Retailers have been looking for a way to automate their workforce for quite some time. From the rapid expansion of self-service checkouts in local supermarkets to more high-end image recognition-powered solutions like Amazon Fresh, automation is very much part of the retail end goal.
However, despite the retail industry gradually advancing towards a fully automated future, there still remain some significant hurdles to overcome along the way. Speed of deployment and the accuracy of current image recognition technology are just two of the biggest issues being faced.
In the retail space in general, Image Recognition (IR) has many applications and advantages, such as on-shelf stock monitoring and auditing. At present, businesses that don’t employ some form of image recognition are more likely to have a number of inconsistencies in their stock.
Meanwhile, businesses that use image recognition tend to be left feeling disappointed that their technology is unreliable and has difficulty differentiating between products on busy shelves.
Often image recognition solutions will struggle to identify products in bad lighting conditions or when a product has been deformed. The general unreliability of existing IR solutions has caused much frustration for Field Marketing Agencies (FMAs), Sales Force Automation (SFAs), and Consumer Packaged Goods (CPGs) companies who rely on IR so they can conduct on-shelf auditing, verify planogram compliance and conduct various data analysis of their products.
How does Image Recognition work?
As the name suggests, image recognition refers to the “recognition” of a product, person, or any other physical object in an image, or video by computer software.

In the retail industry, recognition software is used for several purposes, such as checking stock levels and conducting audits. It can also be applied in self-checkout machines (with IR aiding in loss prevention) or in building autonomous stores (with IR tracking the shopper-shelf interaction). Facial recognition software can even be used for checking shopper IDs for age-restricted goods in some cases, helping give customers a smoother shopping experience. For the purpose of this article, however, we will be focusing on image recognition for retail execution as opposed to facial recognition.
So now that you’re familiar with what image recognition is, it’s a good idea to break down how it all works.
It Starts With Computer Vision?
All image recognition solutions are powered by a type of artificial intelligence (AI) called Computer Vision.
In a similar fashion to human vision, computer vision enables a computer to “see” and contextualise images and videos much in the way our brains and eyes operate. However, unlike humans, computer vision trains machines (machine learning) to process and identify imagery far faster than a human being ever could.
For computer vision to efficiently and accurately detect objects, it requires large amounts of data to be analysed before it can begin to decipher any real-world images. In a retail scenario, this “data” would typically be images of a particular product taken at different angles, in different environments and under multiple lighting conditions. Once this process is complete, a human being then needs to go through all images and provide annotations on the position and class of each product.
Naturally, CPGs face a number of challenges collecting and annotating SKU data to train IR programmes. For example, brands may produce a large number of products. The rate of SKU catalogue change may also be an issue, plus, one product may have varying packaging designs. All of this data needs to be accounted for, but gathering all of this information manually may be exceptionally time-consuming and prone to human error during the annotation process.
Thankfully, there is another way to train a computer vision model which doesn’t require resourcing weeks/months of manual SKU annotation or thousands of real photos. This process is known as synthetic computer vision.
What is Synthetic Computer Vision?
Synthetic computer vision is an alternative approach to computer vision that replaces real data with synthetic data in the training stage of building models/algorithms of retail locations.

Unlike computer vision which requires the painstaking process of collecting real photos of a product from different angles and lighting conditions, synthetic computer vision instead can generate the necessary information that a computer vision model requires entirely from synthetic data.
How does Synthetic data power Synthetic Computer Vision?
As the name suggests, synthetic data is information created synthetically using virtual reality (usually in the form of artificial images or videos) rather than real-world data.
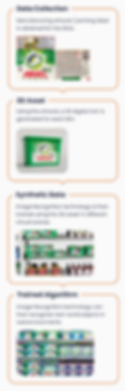
Synthetic data enables a synthetic computer vision model to “learn” with greater accuracy, diversity and at a scale that simply isn’t possible when using real data.
One of the many advantages synthetic data has compared to real data is the fact that synthetic data can align with your expansion plans and not merely deployed as a reactionary procedure. That is to say, the scalability of synthetic data makes it possible for CPGs to conduct image recognition across their entire product line rapidly and across multiple retail locations with ease.
In addition, because synthetic computer vision uses synthetic data, a CPG can conduct image recognition training on their latest products before they have hit store shelves.
Compared to traditional image recognition's reactive methodology, these proactive capabilities mark a revolution. Previously, retailers, FMAs, SFA companies, or CPGs could only train their respective image recognition algorithms after a product had been physically available.
As highlighted above, the improved accuracy of synthetic computer vision models is also one of the significant benefits of this method compared to traditional computer vision. Synthetic data can generate images with specific criteria and properties which simulate in advance many of the possible scenarios that a product will be encountered in the real world (e.g. SKUs on the shelf). This crucial differentiator is arguably the most poignant example of why the future of retail shelf auditing is synthetic.
Neurolabs’ ZIA deploys synthetic data and computer vision, allowing companies to go from a reactionary IR technology solution to a proactive one.
Additionally, the in-store camera or mobile device used by a Field agent may have difficulty recognising products if they are in direct light, or in lighting conditions that differ drastically from the real-world images used to train the image recognition software.
These conditions are virtually impossible to recreate when taking photos in a controlled environment because it would take a considerably long time. Plus, the dataset would be constrained to whatever level of diversity it had acquired up to that point. However, by using a synthetic computer vision-powered image recognition solution such as our ZIA (Zero Image Annotations) technology, it’s possible to replicate such scenarios in a short time frame using virtual scenes that mimic the characteristics of real-life store shelves. Moreover it can be used to simulate scenarios such as products becoming damaged or displaced on the shelf.

The virtual scenes created by our ZIA solution serve as a dedicated “training ground” for the AI so that when faced with similar scenarios in the real world, the technology can detect the products on shelves with a consistent and high level of accuracy, offering CPGs, FMAs and SFAs access to reliable image recognition technology for the first time. Potentially, you could use Synthetic Data to generate unlimited amounts of images with your desired level of diversity.
The future of Retail Shelf Auditing is here
Synthetic computer vision is both the future and natural evolution of retail execution and image recognition.
As AI and machine learning continue to advance, CPGs, SFAs and FMAs that have not integrated a synthetic-based platform into their image recognition solutions will be at a significant disadvantage.
Click below to download our ebook today and get started on your journey to enhanced retail execution.
At Neurolabs, we are revolutionising in-store retail performance with our advanced image recognition technology, ZIA. Our cutting-edge technology enables retailers, field marketing agencies and CPG brands to optimise store execution, enhance the customer experience, and boost revenue as we build the most comprehensive 3D asset library for product recognition in the CPG industry.