Generating virtual products to solve traditional image recognition (IR) problems.
Sagra Technology Struggle to Improve Shelf-Recognition Process of Pharmaceutical Goods in Pharmacies.
At Neurolabs, we believe that a problem is just a challenge that has yet to find its ideal solution. That is why, when the Polish-based tech firm Sagra Technology came to us and said they needed a fast-to-implement solution that would let them upgrade the process of auditing pharmaceutical goods as part of their solution, we went from onboarding to project delivery in just seven days.
Sagra Technology started creating mobile solutions to support sales, marketing and analytics in 1998. With over 34 years in the market they cite their continued success as a matter of speed. They work differently from the trend and are ahead of the market by a technological step.
As an innovative solution provider Sagra recognised the need for a more advanced technology provider, one that could work with them in the challenging pharmaceutical space to significantly reduce their clients' onboarding time as well as delivering just-in-time results of image recognition.
With 25 practically identical products to audit, the only difference being the number of doses in each package, Sagra was facing a challenge: to deliver results directly to sales representatives during their visit to the pharmacy while at the same time achieving the highest level of product detection accuracy.
This is when they turned to Neurolabs, where our synthetic computer vision method overcame the limitations of traditional IR to provide the optimal solution that Sagra was looking for with the project completed in a mere seven days – a fraction of the market standard of a few weeks.

"We have been embedding traditional IR technology in Emigo, our SFA solution for some time, but quickly realised we need to provide results faster and run the IR service for customer in days not months. The time-consuming process of preparing the AI recognition model was not a viable solution for us. We needed a solution that could quickly onboard customers and create models significantly faster but with maintaining in the same time the highest possible accuracy, and of course being able to integrate seamlessly with our Emigo SFA System. Neurolabs' ZIA has been a true game-changer for us. Its synthetic data approach to IR as well as the way of model creation and new SKUs onboarding has revolutionised our operations!"- Sagra Technology
The Synthetic Data Difference
Sagra was interested in our technology but wanted to test it to see if it could deliver results in seconds instead of hours.

With the classic method of IR, you need to collect a painstakingly large amount of real data. Once collected, this real data must then be annotated manually by putting bounding boxes over each product to identify it as a unique SKU.
Once you have gone through the arduous process of collecting several annotated images, you can then feed an algorithm that will then be able to detect the products in new images.
However, at Neurolabs, we do things differently. Our product ZIA (Zero Image Annotation) is built in such a way that we don't annotate real data. Instead, we generate synthetic data and 3D models, allowing for a faster solution that has proven to be more robust and reliable than real data, delivering Sagra Technology 98.3% product detection accuracy. And these results are not atypical for our clients. Our technology achieves an average visual detection accuracy rate of 95% from the outset which then increases to above 98% for specific categories.
To also alleviate any potential anxieties about the effectiveness of our Image Recognition technology, we offer a quality audit service facilitated by an outside, independent provider to prove that the accuracy of the Neurolabs service meets the requirements laid out in the agreement.
Taking real images and annotating them can be costly and time-consuming, resulting in multiple delays depending on the quality and quantity of the captured images.
Not only is it time consuming to collect, but implementing these traditional IR technologies can be painfully slow. Thanks to ZIA, Sagra’s project was delivered in only a week. ZIA is built to onboard quickly, being able to add a CPG customer in as little as one day and achieve a time to market of one week for up to 1000 SKUs.
Integration is another vital tool that allows us to deliver the project so fast. Sagra was able to seamlessly integrate ZIA with its existing solutions and tech stack via cloud APIs.
For Sagra, the Neurolabs’ synthetic approach to IR helped them shift from a reactive to a proactive approach. With ZIA, they could upload new products and changes in packaging before they even hit store shelves, resulting in time-savings and improved operational efficiencies.
A Change-proof AI Recognition Model and Accurate Product Data
One of the struggles that Sagra previously encountered was the process of adding new SKUs and the time-to-learn period that the AI model needs in order to detect new SKUs while maintaining the required accuracy.
When a product is out on store shelves, it is impossible to guarantee that the product will always appear as it does in real data imagery. Depending on the product, there is a likelihood that the item might be picked up, rotated, deformed, opened, or even moved to another shelf with unfavourable lighting.
As the product is rarely found in the same manner as when it was displayed, the traditional or classic approach to IR can struggle to recognise products. Minor differentiations in product packaging also make it difficult or sometimes impossible for traditional IR to distinguish between products. For Sagra, whose 25 SKUs are almost identical, this presented a major challenge. However, for Neurolabs' technology, it was quickly able to distinguish between the products, which only differed in the area of displaying the dose.
Using synthetic data provides the ability for our algorithm to learn from millions of varied positions and angles, guaranteeing a high level of product detection accuracy when field reps are in-store performing shelf audits with their cameras, iPads, smartphones, etc., regardless of the SKUs' placement on the shelf.
Synthetic Computer Vision in Action
To begin, Sagra supplied our team with PDF images of the packaging labels for all 25 SKUs. After we had received the packaging images, we used ZIA to make synthetic 3D assets of the 25 SKUs, which were then tested and trained within a virtual environment.
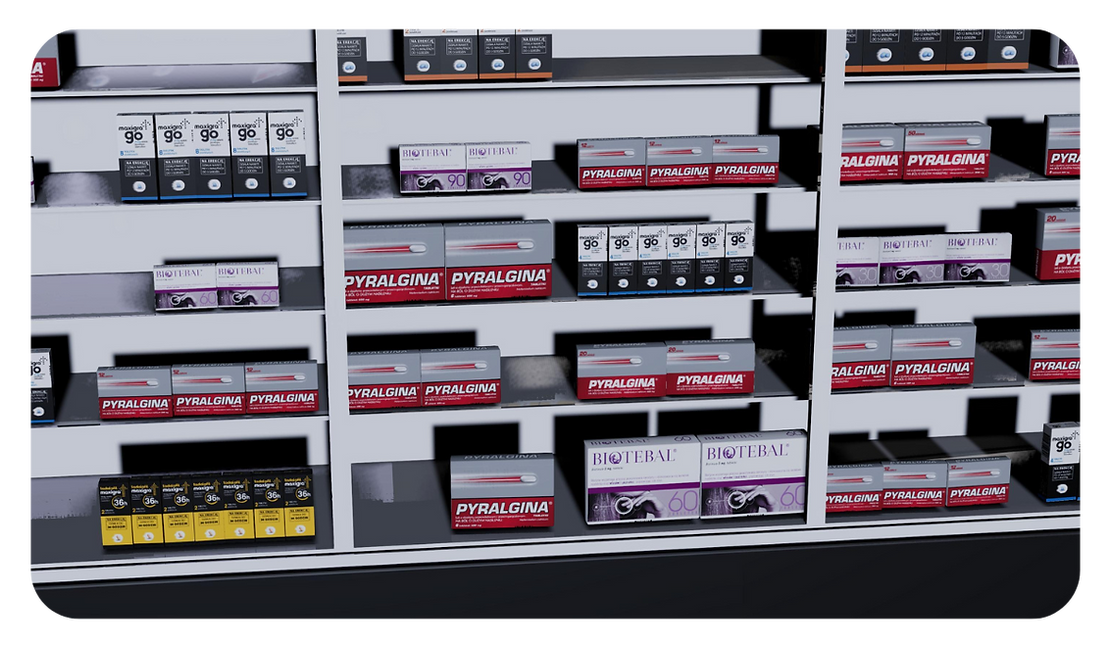
Our testing phase lasted approximately five days, allowing us to extensively train our machine learning algorithm to detect the subtle differences between each label. Once the differences could be distinguished, we were then able to construct auditing scenes using synthetic data.
Tests conducted by Sagra found that our algorithm could successfully detect the products with a 98.3% accuracy whilst delivering end results within 5 seconds on average, far exceeding Sagra’s expectations.
"Neurolabs not only managed to deliver a viable solution to our challenge in an unprecedentedly fast and efficient manner, but they also did so with near-perfect accuracy. We faced some challenges with some pictures (blurs, pictures from above, glares, products covered with shelving elements) but we are well aware of them and together we are working to address them (e.g. providing alternative predictions, improvement of the data collection process)."- Sagra Technology
The Perfect Partner
Following our successful initial project together, we are proud to announce that we will work together alongside Sagra for future endeavours as we collectively aim to improve the execution and speed up the adoption of IR in Poland.
With a specific focus on both the customers of Pharma and Fast-Moving Consumer Goods (FMCG), our joint goal is to build a viable solution that caters to customers of all sizes throughout the respective sectors.
If you're looking for a way to reduce product detection errors in your shelf auditing, Get in Touch with our team and learn how ZIA can easily integrate into your current solution. We'll take you through our technology and show you how it can help.
At Neurolabs, we are revolutionising in-store retail performance with our advanced image recognition technology, ZIA. Our cutting-edge technology enables retailers, field marketing agencies and CPG brands to optimise store execution, enhance the customer experience, and boost revenue as we build the most comprehensive 3D asset library for product recognition in the CPG industry.